What does Edge adaptability actually mean?
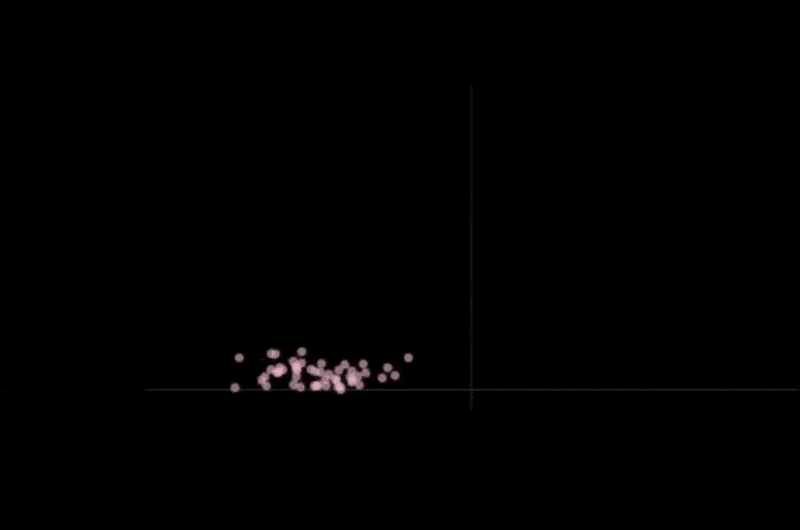
The Need for AGI: The Adaptability Imperative
Artificial Intelligence (AI) is no longer a futuristic concept—it is now deeply embedded in our daily lives. From smartphones and smart home devices to industrial systems and healthcare equipment, AI is everywhere. This widespread adoption signifies a profound shift: AI is no longer confined to controlled environments but is increasingly deployed in dynamic, real-world settings.
However, as AI systems become integral to critical sectors like defense, transportation, public safety, and industrial operations, a glaring limitation has surfaced. While the world around us constantly changes, most AI systems remain static after deployment. This “adaptability gap” is becoming a significant hurdle as we strive to develop more personalised and mission-critical AI applications capable of transforming these sectors:
- Defense: Adversaries continuously evolve their tactics, demanding AI systems that can adapt to new threats in real-time.
- Transportation: Autonomous vehicles and smart traffic systems must navigate unpredictable road conditions and emerging scenarios.
- Public Safety: Criminals and hazards evolve rapidly, requiring AI that can learn and respond to new threats instantly.
- Industrial Parks and Campuses: Dynamic environments with ever-changing equipment, personnel, and threats necessitate AI that can adapt on the fly.
The need for Artificial General Intelligence (AGI)—systems that can learn, reason, and adapt like humans—has never been more urgent.
How Standard Architectures Learn and What They Learn
Traditional AI models, such as Transformers, CNNs, and GNNs, excel at specific tasks by processing large datasets to identify patterns and correlations. However, they rely heavily on historical data and centralized retraining, making them static and resource-intensive after deployment. Traditional AI systems extract general knowledge from datasets by learning features at different levels:
- Low-Level Features: Basic elements like edges, textures, or single words.
- Mid-Level Features: Patterns or structures, such as shapes in images or phrases in text.
- High-Level Features: Abstract concepts like objects in images, sentiment in text, or actionable insights.
By integrating these features hierarchically, AI models generalise patterns for specific tasks
However, once deployed, they cannot adapt to new scenarios without expensive retraining, making them inflexible and ineffective in changing environments. As MIT Technology Review aptly noted, “The way we train AI is fundamentally flawed.”
Real-time On-device Adaptability without training or datasets: What does it mean?
Edge adaptability enables AI systems to learn and adapt in real-time directly on the device, responding to new signals without relying on historical datasets, cloud-based retraining, or large datasets. Inspired by human learning, it achieves generality through a single image or experience—allowing for immediate understanding and adaptation in dynamic, unpredictable environments.
On-device edge adaptability is built on four foundational principles that empower AI to respond effectively in real-time:
- Self-Identify: Detect new events, patterns, or anomalies—including false positives—without requiring prior training.
- Self-Label: Categorize new data or events autonomously, with no human intervention.
- Self-Learn: Continuously adapt and generalize from every single relevant input in real-time, such as a single image or experience, without relying on training datasets or retraining cycles.
- Self-Refine: Consolidate and improve knowledge over time, filtering out noise to maintain accuracy and consistency in decision-making.
These principles enable AI systems to remain dynamic, adaptive, and effective in complex, ever-changing environments.
Skylark Labs’ New Memory System: A Breakthrough in AI
The company has created a revolutionary two-part AI system that combines:
- Core knowledge – the foundation of what the system knows
- Adaptive knowledge – new information it learns over time
Key Features:
The system can:
- Learn and adapt in real-time directly on devices
- Recognize new situations by comparing them to its existing knowledge
- Reduce false alarms effectively
- Learn from just one example and apply that knowledge broadly
- Work without needing large datasets or time-consuming retraining
- Move new learned information into its core memory over time
Conclusion: The Future of AI is on the Edge
Edge adaptability represents a fundamental shift in how we think about AI. It moves us away from static, dataset-dependent systems and toward dynamic, adaptive intelligence that can thrive in an unpredictable world.
By incorporating learning at different temporal scales—short-term, medium-term, and long-term—we can create AI systems that are not only reactive but also proactive, evolving alongside the world they operate in.
As we continue to push the boundaries of AI, one thing is clear: the future of intelligence lies not in the cloud, but on the edge—where learning happens in real-time, in the real world.
Related Blog
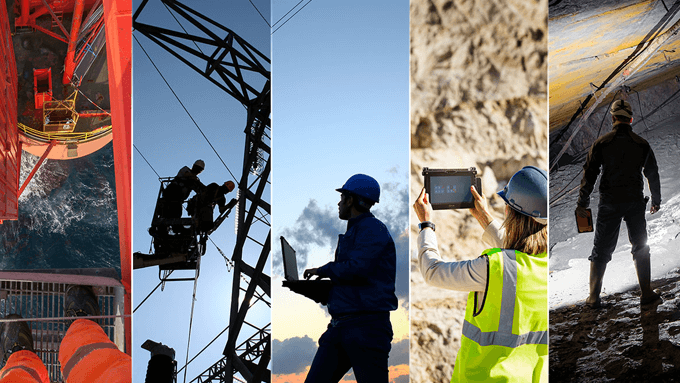
Top 5 Common Safety Incidents in Industries and How Technology Can Prevent Them

Comprehensive Security Solutions for High-Traffic Areas Like Ports and Airports